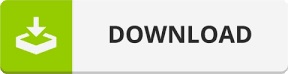
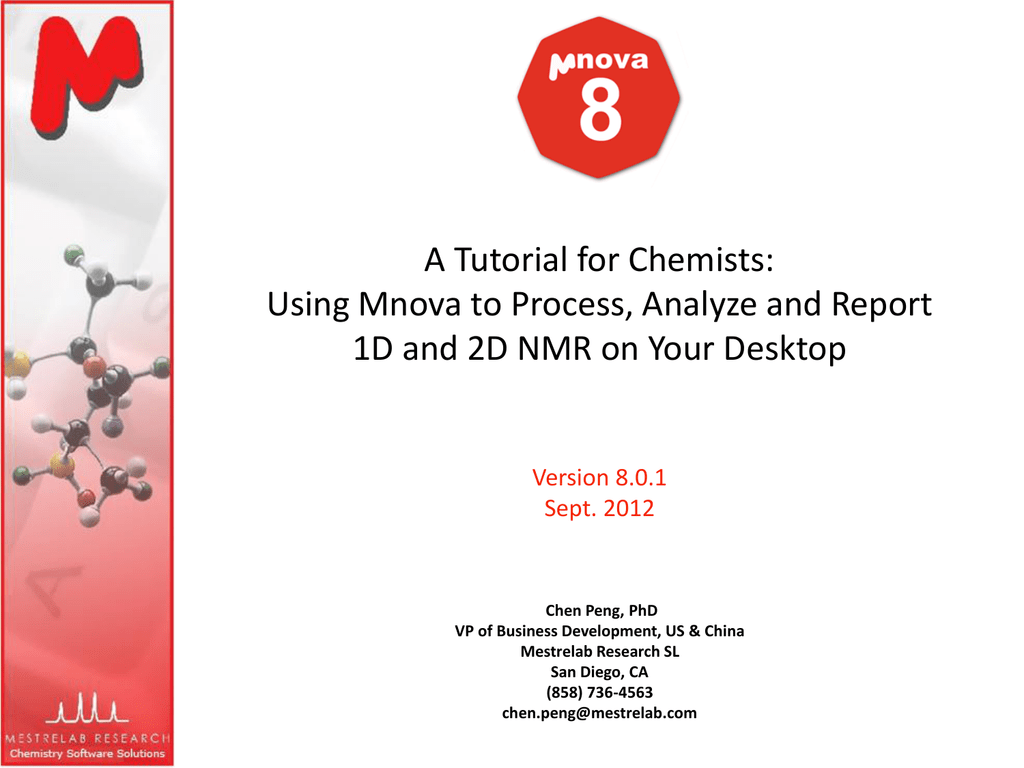
Another approach models spectra as multivariate Gaussian densities followed by filtering with respect to peak intensities and widths 16, 17, 18. Other peak picking methods exploit various forms of matrix factorization 12, 13, 14, or singular value decomposition 15. Early methods focused on criteria based on signal intensity, volume, signal-to-noise ratios, and peak symmetry 3, 4, 5, 6, 7, 8, 9, 10, 11. To address these formidable challenges, numerous approaches have been developed in the past. Moreover, in crowded regions, some peaks may not correspond to maxima because of the close vicinity of larger peak(s) with which such shoulder peaks overlap. However, because of spectral noise, not all local maxima belong to true peaks. The simplest approach is to select local maxima as peak positions. This makes the availability of an approach necessary that accomplishes the above tasks both with high accuracy and high reproducibility.ĭifferent methods have been proposed for peak picking and spectral deconvolution. However, due to the large number of cross-peaks, such work can be tedious, time-consuming, and subjective with results differing between experts and labs, thereby limiting the transferability of the analysis within the research community. This applies in particular to spectra of large molecular systems or complex mixtures containing many cross-peaks that tend to overlap, which makes their spectral deconvolution challenging without expert human assistance. Despite many years of progress, the above steps can only be partially automated. The analysis of an NMR spectrum invariably involves some or all of the following steps: (i) identification of the complete set of cross-peaks, known as peak picking (ii) assignment of each cross-peak to the atoms it belongs to and (iii) quantification of each cross-peak by the determination of the peak amplitude or volume. The parameters that define the cross-peaks represent the chemical and biological information of interest about the molecule(s) present in the sample. frequency coordinates corresponding to chemical shifts), its peak shape along each dimension (usually Voigt shape with variable amounts of Lorentzian or Gaussian components), and its peak amplitude (or volume). Each cross-peak is characterized by the position of its center (i.e. Identification and quantitative characterization of cross-peaks critically affect all downstream analyses and can have a major impact on data interpretation. A spectrum can consist of several hundred to thousands of cross-peaks manifested as localized multidimensional spectral features that in the case of 2D NMR belong to individual pairs of atoms that possess a nuclear spin. Multidimensional NMR spectroscopy is a powerful and versatile method for the quantitative characterization of a wide range of molecular systems ranging from small molecules to large biomacromolecules and their complexes 1, 2. DEEP Picker should facilitate the semi-automation and standardization of protocols for better consistency and sharing of results within the scientific community. We demonstrate the utility of DEEP Picker on NMR spectra of folded and intrinsically disordered proteins as well as a complex metabolomics mixture, and show how it provides access to valuable NMR information. We show that our method is able to correctly identify overlapping peaks, including ones that are challenging for expert spectroscopists and existing computational methods alike. DEEP Picker includes 8 hidden convolutional layers and was trained on a large number of synthetic spectra of known composition with variable degrees of crowdedness. Here, we introduce DEEP Picker, a deep neural network (DNN)-based approach for peak picking and spectral deconvolution which semi-automates the analysis of two-dimensional NMR spectra. The analysis of nuclear magnetic resonance (NMR) spectra for the comprehensive and unambiguous identification and characterization of peaks is a difficult, but critically important step in all NMR analyses of complex biological molecular systems.
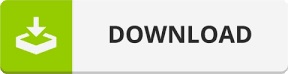